Session Track
November 03 (Friday)
신뢰성부문 포스터
Poster,
3층 로비,
14:10~15:00
- 손영갑(안동대), 오현석(광주과기원)
Fr16D-1
14:10~15:00
고장예지를 위한 기계학습 데이터 전처리 기법 개발
Many mechanical structures are failed because they subjected to repeated random loading. They are used for a specified period to prevent damage from sudden failure. Therefore, even if there is no fails until end of specified lifetime, it should be replaced. If it is possible to predict the failure according to the actual environment, stability and efficiency can be increased. In this study, failure of a shackle, which is used to connect the cargo in the hoist efficiently, is predicted under the repeated loading. Data preprocessing, simple machine learning method, and logistic regression were used. Preprocessing includes normalization and linearization to decrease learning time and make easy to control prognostic distance. With preprocess and learned decision boundary line, it averagely predicted failure at 97.3% of crack detected time.
Keywords : PHM(고장예지기술), Machine Learning(기계학습), Shackle(샤클), Preprocess(전처리)
Paper : Fr16D-1.pdf
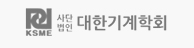
(사)대한기계학회, 서울시 강남구 테헤란로 7길 22 한국과학기술회관 신관 702호, Tel: (02)501-3646~3648, E-mail: ksme@ksme.or.kr