Session Track
November 03 (Friday)
신뢰성부문 포스터
Poster,
3층 로비,
14:10~15:00
- 손영갑(안동대), 오현석(광주과기원)
Fr16D-11
14:10~15:00
인공지능 생성모델 활용 공학시스템 센서 데이타 모방 재현: 개념적 접근
Fault diagnosis is a critical research topic to ensure the reliability of safety-related systems. For fault diagnosis, data-driven approaches have received a significant amount of attention from the academia and industry due to advantages over physics-based approaches as the data-driven approaches require neither understanding complex failure mechanisms nor developing expensive life models. Nevertheless, the development of data-driven approaches is still challenging. One of the main reasons is the dearth of sensor data from engineered systems, in particular, faulty data. To this end, this study attempts to emulate data using a generative model of artificial intelligence. The generative model consists of two parts: generator and discriminator nets. A case study with MNIST database is conducted to demonstrate the effectiveness of the proposed model. We expect that this study can be extended with real data from power plant systems such as turbines, generators and pumps.
Keywords : Sensor signal(센서신호), Faulty data(고장데이터), Generative model(생성모델), Artificial intelligence(인공지능), Deep learning(딥러닝)
Paper : Fr16D-11.pdf
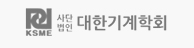
(사)대한기계학회, 서울시 강남구 테헤란로 7길 22 한국과학기술회관 신관 702호, Tel: (02)501-3646~3648, E-mail: ksme@ksme.or.kr