Session Track
November 02 (Thursday)
일반기계 및 부품 신뢰성(III)
Oral,
제9발표장(303A호),
08:40~09:40
- 오현석(GIST)
Th09A-4
08:40~09:40
데이터 불균형 문제를 해결하기 위한 Generative Adversarial Networks (GAN) 기반의 가상 데이터 합성
With the development of manufacturing technology and the introduction of the smart factory, the productivity and the
amount of data to be acquired has significantly increased Recently, deep learning has been used to replace the existing
machine learning algorithms with improved predictive performances. With sufficient amount of data, solving
classification problems using the deep learning model can approximate any underlying probability. However, a typical
class imbalance in manufacturing field harms performance of the deep learning models. Although methods such as
resampling or reweighting data have been proposed to solve the data imbalance issue, such methods are not considered
as a fundamental solution to the issue. In this paper, we propose the Generative Adversarial Networks (GAN) which can
learn the distributions of a real data set, and generate a virtual dataset that will be utilized for numerous manufacturing
applications. To verify the proposed approach, we compared the performances of a model trained with the imbalanced
dataset and another model trained with data generated by GAN.
amount of data to be acquired has significantly increased Recently, deep learning has been used to replace the existing
machine learning algorithms with improved predictive performances. With sufficient amount of data, solving
classification problems using the deep learning model can approximate any underlying probability. However, a typical
class imbalance in manufacturing field harms performance of the deep learning models. Although methods such as
resampling or reweighting data have been proposed to solve the data imbalance issue, such methods are not considered
as a fundamental solution to the issue. In this paper, we propose the Generative Adversarial Networks (GAN) which can
learn the distributions of a real data set, and generate a virtual dataset that will be utilized for numerous manufacturing
applications. To verify the proposed approach, we compared the performances of a model trained with the imbalanced
dataset and another model trained with data generated by GAN.
Keywords : Generative Adversarial Networks (GAN), Data Imbalance (데이터 불균형), Data Generation (데이터 생성), Deep Learning (딥러닝), Machine Learning (기계학습).
Paper : Th09A-4.pdf
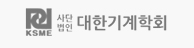
(사)대한기계학회, 서울시 강남구 테헤란로 7길 22 한국과학기술회관 신관 702호, Tel: (02)501-3646~3648, E-mail: ksme@ksme.or.kr