Session Track
November 01 (Wednesday)
[특별세션] 계간축열(I)
Oral,
제2발표장(201B호),
13:30~14:30
- 조홍현(조선대)
We02A-2
13:30~14:30
에너지 효율 예측을 위한 점증적인 지능모델의 설계
In this study, we introduce and discuss a concept of an incremental RBFN model. In contrast to
typical rule-based systems encountered in fuzzy modeling, the underlying principle exploited here is to
consider a two-phase development of fuzzy models. First, we build a standard regression model which
could be treated as a preliminary construct capturing the quadratic polynomials part of the data and
in this way forming a backbone of the entire construct. Next, all modeling discrepancies are
compensated by a collection of rules that become attached to the regions of the input space in which
the error becomes localized. The incremental model is constructed by building a collection of
information granules through some specialized fuzzy clustering, called context-based (conditional) Fuzzy
C-Means that is guided by the distribution of error of the quadratic polynomials part of the model.
The architecture of the model is discussed along with the major algorithmic phases of its development.
In particular, the issue of granularity of fuzzy sets of context and induced clusters is discussed
vis-à-vis the performance of the model.
typical rule-based systems encountered in fuzzy modeling, the underlying principle exploited here is to
consider a two-phase development of fuzzy models. First, we build a standard regression model which
could be treated as a preliminary construct capturing the quadratic polynomials part of the data and
in this way forming a backbone of the entire construct. Next, all modeling discrepancies are
compensated by a collection of rules that become attached to the regions of the input space in which
the error becomes localized. The incremental model is constructed by building a collection of
information granules through some specialized fuzzy clustering, called context-based (conditional) Fuzzy
C-Means that is guided by the distribution of error of the quadratic polynomials part of the model.
The architecture of the model is discussed along with the major algorithmic phases of its development.
In particular, the issue of granularity of fuzzy sets of context and induced clusters is discussed
vis-à-vis the performance of the model.
Keywords : radial basis function networks, linear regression, quadratic polynomial, predictor
Paper : We02A-2.pdf
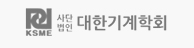
(사)대한기계학회, 서울시 강남구 테헤란로 7길 22 한국과학기술회관 신관 702호, Tel: (02)501-3646~3648, E-mail: ksme@ksme.or.kr