Session Track
November 02 (Thursday)
인공지능/머신러닝(II)
Oral,
제13발표장(401B호),
11:00~12:00
- 오현석(GIST)
Th13C-2
11:00~12:00
Deep Neural Network for Fault Diagnosis of Power Transformers using Dissolved Gas Analysis
The dissolved gas analysis, produced by deterioration of insulating oil, is the most popular diagnostic tool to detect various incipient faults in power transformers. So far, the handcrafted DGA features, such as DGA composition ratios(i.e., C2H2/C2H4, C2H4/C2H6, CH4/H2), have been often used as the input features of shallow learning or used to identify diagnostic criteria (i.e., Dornenburg Ratio, Rogers Ratio, IEC ratio) for the fault diagnosis of power transformers. However, a false alarm rate is relatively large due to the limitations of the handcrafted features because they are made up of gas combinations that can classify the fault types in a low dimensional space that can be classify the fault types in a low dimensional space that can be analyzed by the human inspection. To enhance DGA-based diagnostic accuracy, a novel method using deep neural network (DNN) is proposed to determine high-level features without relying on the handcrafted features. Specifically, many layers of nonlinear unit in a DNN convert the raw DGA data into discriminative representation without losing high-dimensional information.This makes health classification more effective. A proposed method is validated from the reference database of IEC TC 10, which is the visual inspection data of transformer faults. The results indicate that the proposed DNN approach achieves higher accuracy than the existing methods based on shallow learning with the hancrafted features.
Keywords : Deep Neural Network, Dissolved Gas Analysis, Power Transformer
Paper : Th13C-2.pdf
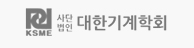
(사)대한기계학회, 서울시 강남구 테헤란로 7길 22 한국과학기술회관 신관 702호, Tel: (02)501-3646~3648, E-mail: ksme@ksme.or.kr