Session Track
November 02 (Thursday)
인공지능/머신러닝(II)
Oral,
제13발표장(401B호),
11:00~12:00
- 오현석(GIST)
Th13C-3
11:00~12:00
Convolutional Neural Network를 이용한 저널베어링 로터시스템 고장 진단
In order to enhance the reliability and performance of journal bearing rotor systems, some fault diagnosis methods using several types of sensor signals have been proposed. In these data based diagnosis methods, feature extraction for devising and extracting proper features is very important step. However, this procedure is time-consuming and requires lots of physical/domain knowledge about target systems. Furthermore, unless the appropriate features, which can represent the states of systems well, are extracted, the performance of those methods cannot be guaranteed. To solve those difficulties, novel diagnosis technique using deep learning is proposed in this paper.
In this research, vibration data is acquired from journal bearing rotor systems via proximity sensors at first. In conventional rotor systems, two proximity sensors are installed in perpendicular position to acquire vibration signals. In this work, using vibration signals acquired from those two sensors, omnidirectional regeneration (ODR) technique is applied to obtain additional virtual sensor signals along the circumference. Then using those ODR signals, vibration images are generated. After that, using convolutional neural network (CNN); a kind of deep learning algorithm which is very powerful in image recognition tasks, the optimal features can be extracted automatically from those images. By comparing the class prediction accuracy, we can validate the performance of proposed novel diagnosis technique using CNN.
In this research, vibration data is acquired from journal bearing rotor systems via proximity sensors at first. In conventional rotor systems, two proximity sensors are installed in perpendicular position to acquire vibration signals. In this work, using vibration signals acquired from those two sensors, omnidirectional regeneration (ODR) technique is applied to obtain additional virtual sensor signals along the circumference. Then using those ODR signals, vibration images are generated. After that, using convolutional neural network (CNN); a kind of deep learning algorithm which is very powerful in image recognition tasks, the optimal features can be extracted automatically from those images. By comparing the class prediction accuracy, we can validate the performance of proposed novel diagnosis technique using CNN.
Keywords : Fault Diagnosis (고장 진단), Rotor (로터), Journal Bearing (저널베어링), Vibration Signal (진동 신호), Deep Learning (딥러닝)
Paper : Th13C-3.pdf
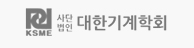
(사)대한기계학회, 서울시 강남구 테헤란로 7길 22 한국과학기술회관 신관 702호, Tel: (02)501-3646~3648, E-mail: ksme@ksme.or.kr